SERVICE|Advanced research "Reliable AI"
To create safe and secure society through artificial intelligence (AI)
We verify the safety of AI systems.
Patent Nr.7007529
"Safety verification system, safety verification method,
and safety verification program
for artificial intelligence system"
We verify the safety of AI systems with a brandnew and patented method (Patent Nr.7007529). The method has been developed by integrating a method of Resilience Engineering: FRAM (Functional Resonance Analysis Method) and one of the formal methods known as SpecTRM-RL (Specification Tools and Requirement Methodology-Requirement Language). It enables us to evaluate the reliability of the AI system in terms of 1) how exhaustive the target AI system is tested and 2) how consistent the AI functions for a given input.
We solve the following issues.
-
Verification of advanced technolog
(e.g., autonomous driving or automatic delivery).Transportation
Automobile
IT
-
Safety analysis of "blackbox" AI systems to make them explainable.
IT
Automatic driving
Application
-
Personal training for the safety and development assurance.
IT
Application
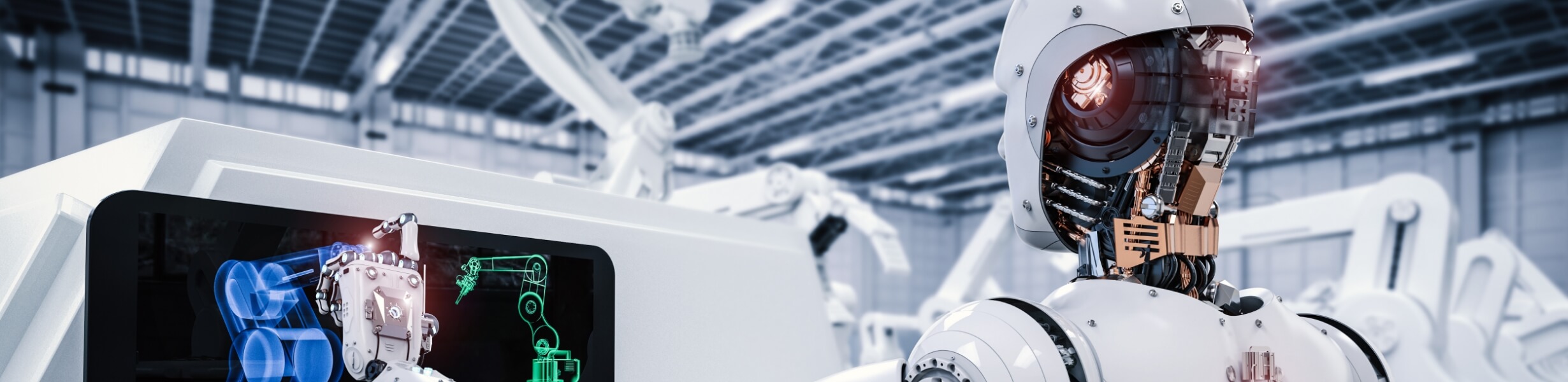
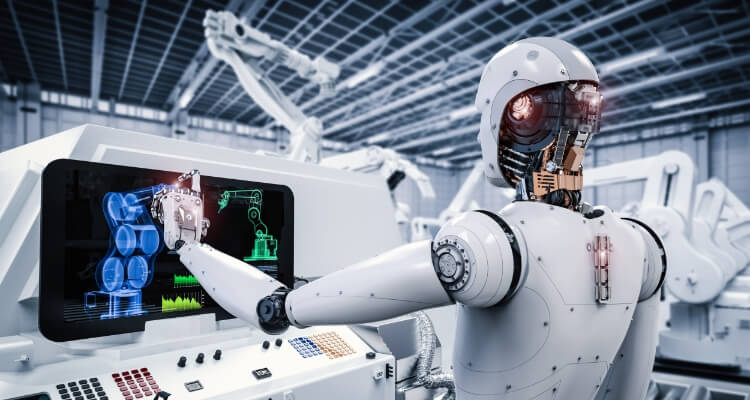
Flow Verification process
Visualization of AI systems for Completeness/Consistency analysis
-
STEP 01
Visualization of
artificial intelligence (AI) systemWe use FRAM to clarify "why AI can solve problems well."
-
STEP 02
Completeness/Consistency
analysis of the visualized AI modelWe perform completeness/consistency analysis of the AI system as a FRAM model by using SpecTRM-RL.
Visualization of AI
The process starts from clarifying “why the target AI system can solve problems well” by using FRAM. FRAM is a method to build a model of how the target system is functioning, and the model is represented as a network of characteristic functions; funcitons in FRAM are defined with six aspects as shown in the following table, and that is why they are viaually represented as hexagons. Those aspects of each function consequently generate complex dependencies among functions, and moreover, a FRAM model as a network of the FRAM functions. The FRAM model analysis provides useful information about how the target system is actually functioning.
Class ification |
Six aspects of function | ||||
---|---|---|---|---|---|
Input | Trigger | Input | |||
Pre condition |
Precondition | ||||
Post condition |
Condition that continuously changes output | Control parameter | Control | ||
Condition that stops output (Output stops due to depletion) | Environment condition | Resource | Resource | ||
Time condition | Time limit | Time | |||
Output | Output |
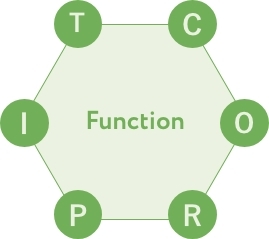
Let's take an example of an AI system classifying passenger cars and trucks from images. The purpose of this visualization process is to identify how the natural intelligence, or in other words, we --- human beings distinguish the difference between those cars and develop a model of their logical structure. In this phase, the methodology of FRAM plays an important role in identifying the essential parameters effectively and exhaustively. The analysis of this example suggests that "cars with 1) cargo bed, 2) roof, and 3) a long nose should be trucks rather than passenger cars, and the conditions 1 -- 3 are therefore the essential parameters for this classification task by the natural intelligence."
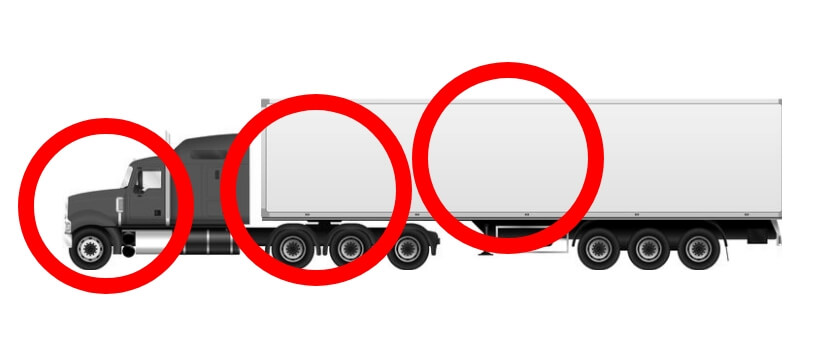
Completeness/Consistency analysis of a visualized AI model
The next step after the FRAM visualization is the completeness/consistency analysis. The
completeness analysis identifies parameters uniquely used for the classification logicl by the
target AI system and their combinations that are still not tested. For instance, the AI system
could use the parameters that we --- human beings did not expect and make decisions based on
such parameters; the AI systems could also provide inconsistent results with respect to an
identical input, depending on the case. The repetition of the completeness/consistency analysis
enables us to find out and fix such imcompletenesds and inconsistency of the AI systems.
Figure 3 shows an example result of the completeness/consistency analysis. The result:
"***INCOMPLETE***" provides the combinations of parameters that are still not tested as a result
of completeness analysis; the TF in the matrix represents which part of a target car (e.g.,
wheel, shape of body, or window) is focused (True) and not focused (False) by the AI. The
result: "***INCONSISTENT***" also shows the combinations of prameters which could result in
inconsistent behavior of the AI system; the AI system could provide inconsistent results if no
parts of a target car are focused by the system since the combination of parameters consists of
only "F" in this case. The process loop of training, FRAM visualization, and
completeness/consistency analysis will go onuntil such incomplete/inconsistent conditions are
solved, and it significantly enhance the reliability of the AI system.
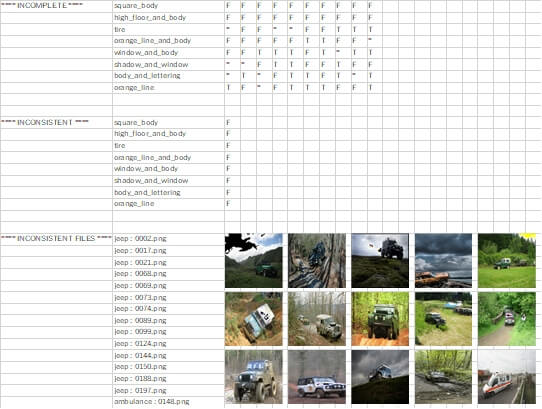
The verification of AI safety makes it possible not only to visualize the process of AI systems but also to provide unforeseen knowledge obtained by the AI systems. This insight suggests that we --- human beings can also learn from the AI systems and utilize the knowledge for the future. It is essential for us to actively interact with the AI systems and find out their success factors. We, JAMSS, believe that such mutual interaction between human and technology, including the AI systems, shall lead us to further development of safe and secure society in the future.
Safety verification of error detection/
malfunction sign detection
AI model
This methodology can also be applied to various data formats, which is not limited to image data. It has been applied to an AI system detecting anomaly signs of space systems since 2019, for instance. In this case, the decision process of the AI system and reasons for their anomaly detection were visualized; the analysis result found that such information can improve the situation awareness of the gournd controllers and support their decision making.
CONTACT
Inquiries
Please use our inquiry form for any question.
Please feel free to contact us.
If you are in hurry, please contact us via phone:
Japan Manned Space Systems Corporation Marketing and Contract Department
Tel: +81-3-3211-2060
(Between 10AM and 5PM on weekdays)